What is Master Data Management?
Author: Lochan Narvekar . February, 04, 2015
Reprinted from 2009 original article by author
Master Data Management is really a business problem that is Age-Old. How do I get best out of my business processes?
But to answer this question one should get the underlying data as well in order! I like to quote, “Quality processes need quality data, but also, vice-versa”. By data I refer to transactional as well as Master data. By consequence, transaction data will depend heavily on the base master data. If there is confusion on master data, how I can guarantee my transaction data to my business? As you can see, this is really an old problem and hence there is multitude of solutions over last so many years. In this article, I will focus on MDM principles I have developed after years of work in this area. There is always scope for improvement, but objective of this article is to set formal base line for understanding MDM scope.
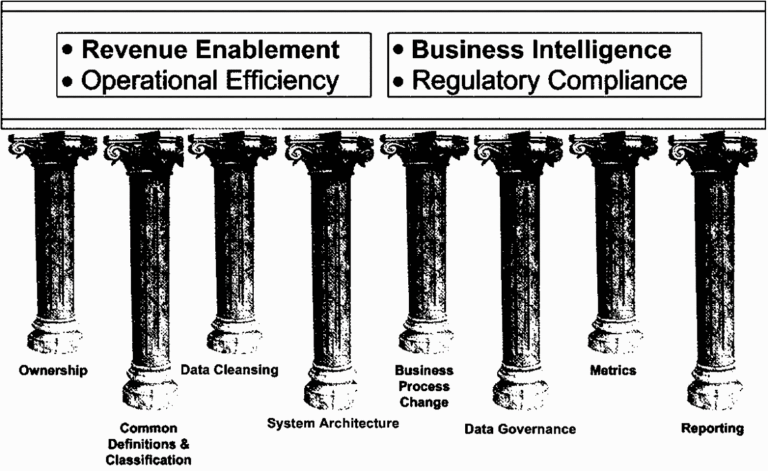
Pillars of MDM
Common MDM Concepts:
Let’s look at each area,
Ownership
After we scope the MDM effort, we will arrive at a set of attribute groups that are going to be under MDM control. It’s essential to set Ownership of these attributes. Meaning which Roles are accountable for the values in these attributes? This helps in getting data cleansed before the Go Live. Also, it helps after go live in making these Roles accountable for accuracy of these attributes.
Common Definitions & Classifications
An attribute called one thing in one system should not be called as something else in other system. This seems like a common sense, but where each system has different organic growth, different owners, this is bound to happen without MDM understanding. MDM helps us tackle this issue from top down.
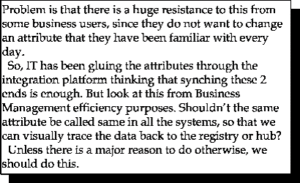
We also need to look at the classification for data element as it drives the attributes needed for that data element. Each classification has different required attributes. As much as possible we need to match the classification across business systems.
E.g. Products are classified as product types in an Engineering system. We must strive to commonize the same product types in Marketing system with same names. There might be more classifications in Marketing and more attributes, but common ones need common names
Logical Data Models are great aid in documenting anomalies and flow of data between systems.
Data Cleansing
Data cleansing has 2 phases to it. One, before Go Live and another, after Go Live. It has surprised me to see that often folks miss on the latter. Imagine cleaning the data, transforming it and after Go Live, it again gets dirty. This problem can be solved with following approach.
First, get a good data cleansing tool and implement it alongside the MDM tool. If the data is small then, manual effort is good enough.
Second, we need to put checks in place to not allow creation of bad data going forward. Treatment here is different for internal vs. external applications.
Third, we have to devise a way to clean the data on on-going basis with Data Stewards, and reports.
MDM Systems and Integrations
This is the most important pillar. Management needs to study the current application set and decide if the organization needs and/or is ready for a new MDM application. Lot can be achieved without implementing a new tool, agreed! But this needs a strong adherence to MDM principles, and a good consultant. I have often reconfigured the existing toolset and with help of MDM principles tightened the business area in question. But, having a specialized tool for especially if the scope is big enough generates good ROI.
Many companies have Enterprise Integration platforms or Enterprise Bus. They play key role in MDM projects tying together the MDM tool and/or all the systems through MDM principles. Newer platforms even offer embedded Business Process Managers to weave the tools together. Remember to look at all of this through MDM lenses as described here.
Business Process Change
As I wrote above, “Quality processes need quality data, but also, vice-versa”. What this means to me is, there is a business and IT partnership in MDM success. What good is the data if the processes are allowing data to change uncontrollably? This change is not drastic. Few checks and balances in the process based on MDM principles to make sure that data stays good.
Data Governance
This is another important pillar! Good data is only going to stay good, if the good data governance is put in place.
This begins with the Attribute Owner. Who is ultimate authority on this attribute (perhaps more adequately, this business sub-function)?
Then, who can see the attribute or group of attributes? Who can change them? Who needs to be notified of the change? This is most important topic and one needs to use templates to aid in this. Who needs to subscribe to the change? What systems need this data? All of these questions need to be addressed.
Metrics and Reporting
We need to put together right metrics so that after go live we can measure the performance of our MDM implementation. And we need reports on top of these metrics.
Author: Lochan Narvekar . February, 04, 2015
Praedicere is a MDM and DG strategy, and IT implementation consulting firm based in California. We work with mid-to large corporations throughout US, including fortune 500 clients.
Lochan Narvekar is the Founder and Principal at Praedicere and has published many articles on the broader topic of MDM and DG